Harnessing Machine Learning for Malware Detection: A New Era in Cybersecurity
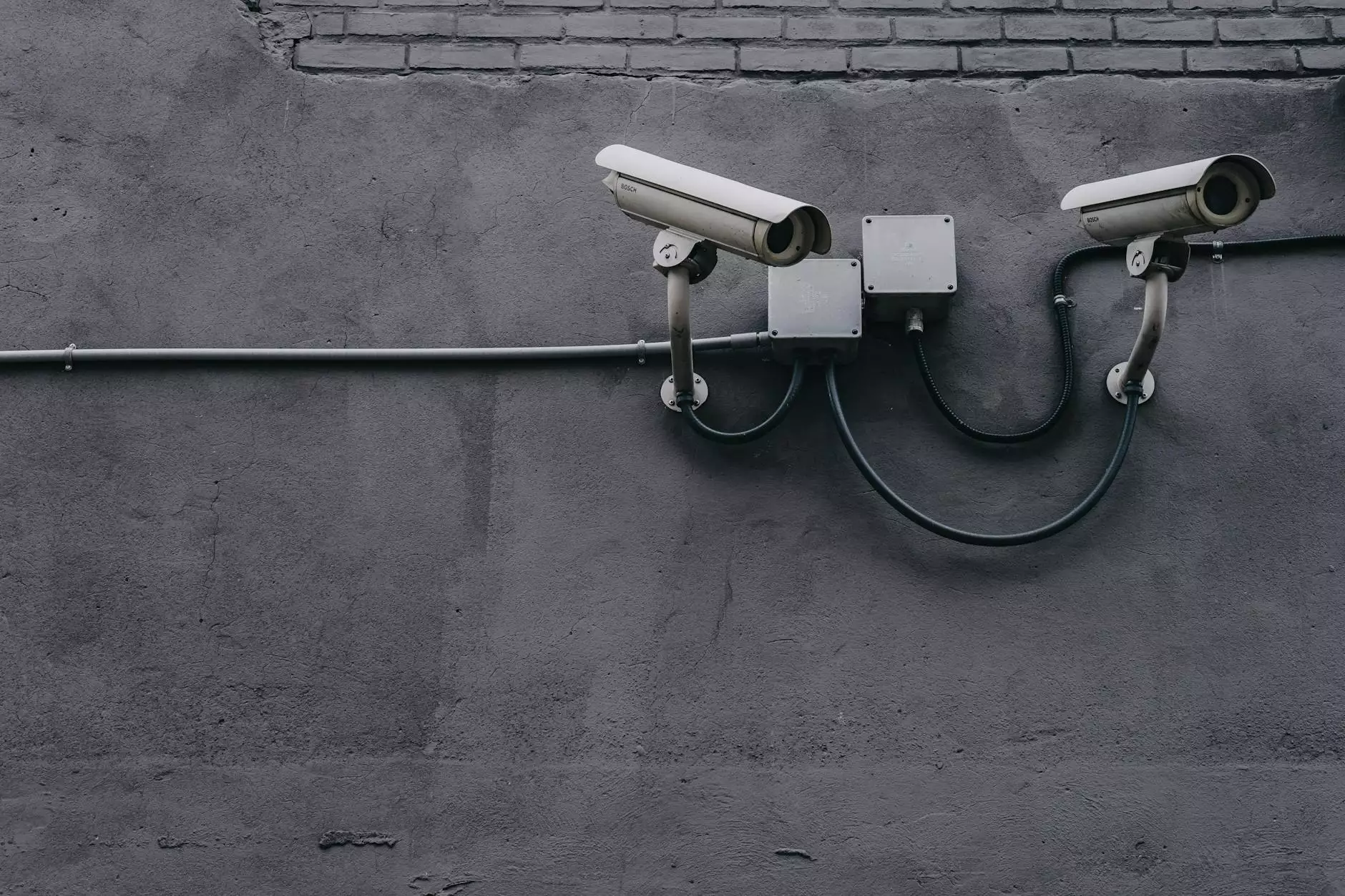
In a world where digital threats are becoming increasingly sophisticated, businesses must adopt advanced technologies to protect their sensitive data. One of the most promising innovations in the field of cybersecurity is machine learning for malware detection. This article delves into how businesses, especially those operating in the realms of IT services and computer repair, can leverage machine learning to identify and mitigate malware threats effectively.
What is Malware?
Malware, short for malicious software, is a blanket term for any software intentionally designed to cause damage to a computer system, server, or network. This includes viruses, worms, Trojan horses, and ransomware. The impact of malware can be devastating, leading to significant financial loss and damaged reputations. Thus, proactive measures are crucial for businesses to protect themselves.
The Necessity of Advanced Detection Techniques
Traditional cybersecurity measures often rely on signature-based detection methods, which can be outdated by the time a threat is identified. In contrast, machine learning provides a dynamic approach that allows for real-time threat detection. This technology continuously learns from new threats, adapting its algorithms to identify potentially malicious activities before they can cause harm.
Understanding Machine Learning
Machine learning (ML) is a subset of artificial intelligence that focuses on building applications that can automatically learn and improve from experience without being explicitly programmed. It allows systems to analyze vast amounts of data, recognize patterns, and make recommendations or decisions based on historical information. In the context of *malware detection*, ML can be utilized to enhance cybersecurity strategies significantly.
How Machine Learning Enhances Malware Detection
1. Pattern Recognition
One of the foremost abilities of machine learning systems is their capacity for pattern recognition. These systems can analyze historical data to recognize indicators of malicious behavior. By learning from previous malware attacks, ML algorithms can identify common characteristics and alert systems to new, potentially harmful software.
2. Anomaly Detection
Machine learning excels at anomaly detection. By establishing a baseline for normal operations within a network, ML models can detect deviations from this norm that may suggest a malware intrusion. This method is particularly effective against zero-day exploits, which are vulnerabilities that have not yet been identified or patched by security experts.
3. Automating Responses
Another advantage of incorporating machine learning in malware detection is the ability to automate responses to threats. Once a potential malware threat is identified, the system can automatically quarantine affected files, block malicious IP addresses, or alert cybersecurity personnel. This swift action can greatly minimize the damage caused by cyber threats.
4. Reduced False Positives
Machine learning can significantly reduce the rate of false positives in malware detection. Traditional systems often trigger alerts for benign software, creating alarm fatigue amongst security teams. With ML, the accuracy of identifying actual threats improves, allowing teams to focus on real threats rather than wasting time on harmless activities.
Implementing Machine Learning for Malware Detection
For businesses, especially those in the security systems sector like spambrella.com, implementing machine learning for malware detection involves several critical steps:
1. Data Collection and Management
The first step is to gather and manage data effectively. Data can include:
- Historical malware samples
- Logs from network traffic
- User behavior data
- Endpoint security reports
By compiling a robust dataset, organizations can train their machine learning models to recognize a wide array of malware behaviors.
2. Choosing the Right Algorithms
Selecting the appropriate machine learning algorithms is crucial for effective malware detection. Popular algorithms for this purpose include:
- Decision Trees
- Random Forests
- Support Vector Machines (SVM)
- Neural Networks
Each algorithm comes with its strengths, and the choice often depends on the specific needs of the business and the complexity of the data.
3. Continuous Training and Updating
Machine learning models require continuous training and updating to remain effective against evolving malware threats. Businesses must regularly feed new data into their models and refine algorithms based on recent malware trends and behaviors to maintain a strong defense.
Benefits of Machine Learning in Business
Integrating machine learning for malware detection comes with numerous benefits for businesses:
1. Enhanced Security
The most significant advantage is undoubtedly enhanced security. As cyber threats evolve, businesses that utilize ML-based solutions can stay ahead of potential malware threats and secure their networks more effectively.
2. Cost Efficiency
By reducing the likelihood of successful malware attacks, businesses can save substantial costs associated with data breaches, including regulatory fines, loss of customer trust, and remediation expenses.
3. Improved Operational Efficiency
Automated responses to threats improve operational efficiency, enabling security teams to focus on strategic initiatives rather than spending excessive time on investigating false alarms.
4. Competitive Advantage
Businesses that adopt cutting-edge technology like machine learning for malware detection can position themselves as industry leaders, attracting clients who value innovation and security.
Challenges of Implementing Machine Learning
While machine learning offers transformative benefits, businesses must also navigate certain challenges:
1. Data Quality and Quantity
Machine learning models require high-quality input data. Poor data quality can lead to inaccurate predictions, resulting in vulnerabilities rather than safeguards.
2. Expertise and Resources
Developing effective machine learning solutions requires a team with expertise in both cybersecurity and data science. Many organizations struggle to acquire or develop this dual expertise.
3. Integration with Existing Systems
Implementing machine learning solutions into existing security frameworks can be complex and may require significant investment in both time and resources.
Conclusion
The integration of machine learning for malware detection represents a significant evolution in the cybersecurity landscape. For businesses focused on IT services and computer repair, leveraging ML technology enhances malware detection capabilities, reduces attacks, and improves overall security posture. Although challenges exist, the investment in machine learning can lead to substantial long-term benefits, making it an essential consideration in today’s digital age.
As organizations like spambrella.com continue to innovate in the realm of cybersecurity, staying abreast of these technological advancements will be critical to protecting sensitive data and sustaining business success. Embracing machine learning is no longer an option but a necessity for proactive cybersecurity strategy.